In the ever-expanding realm of data, a significant portion remains unstructured, posing challenges for traditional database systems. With an astounding amount of unstructured data worldwide, estimated to be 80% of all the data we produce, organizations are realizing the pressing need for advanced solutions that can effectively harness this wealth of information. As artificial intelligence (AI) continues its rapid progress, the ability to extract insights from unstructured data becomes paramount. This is where Vector Databases come into play.
In this blog post, we will embark on a journey to understand Vector Databases. This is an innovative approach to data management designed to address the complexities of unstructured data. As we explore the realm of Vector Databases, we will draw parallels and distinctions with the widely used and familiar Snowflake Cloud Database.
By shedding light on the staggering amount of unstructured data worldwide and its significance for AI-driven advancements, we will lay the foundation for comprehending the necessity of Vector Databases. Through concise explanations and thoughtful comparisons, we aim to provide a high-level introduction to this cutting-edge technology and its relevance in shaping the future of data analytics.
So, let’s dive into the world of Vector Databases—a powerful tool poised to unlock the potential hidden within vast unstructured datasets, propelling us further along the path of AI progress.
How does it work?
At the heart of Vector Databases lies a transformative concept that enhances data processing capabilities: vectorized query execution. Unlike traditional row-based query processing, where each row is processed individually, vectorized query execution operates on batches of data called vectors.
To grasp this concept, let’s consider a simplified example. Imagine a traditional database processing a list of employees and their corresponding salaries. In a row-based approach, the database would examine each employee’s salary individually, one row at a time. On the other hand, a Vector Database would process multiple employee salaries together as a vector. This means, performing computations on them simultaneously. This parallel processing drastically improves efficiency and speeds up query execution.
In essence, Vector Databases harness the power of columnar storage. Columnar storage means, storing data by columns rather than by rows. By organizing data in this manner, the databases achieve several advantages. Firstly, columnar storage allows for efficient compression, reducing storage space requirements. Secondly, it enables selective column access, meaning that only the relevant columns are accessed during query execution. This in turn is minimizing data retrieval and improving performance.
Additionally, databases based on vectors leverage a technique called SIMD (Single Instruction, Multiple Data). SIMD allows the processor to execute a single instruction on multiple data elements simultaneously, further enhancing query execution speed.
Through vectorized query execution, columnar storage, and SIMD, Vector Databases revolutionize the way data is processed. These attributes make them ideal for handling vast amounts of unstructured data and accelerating analytical workloads. In the next section, we will explore the intricacies of columnar storage and its impact on data retrieval and query performance.
Use Cases
Vector Databases shine in various domains where efficient data processing and analytics are crucial. Let’s explore some compelling use cases where the unique capabilities of Vector Databases truly make a difference.
- Real-time Analytics: Vector Databases excel in scenarios that demand rapid analysis of streaming data. Whether it’s monitoring financial markets, analyzing sensor data from IoT devices, or processing social media feeds in real-time, Vector Databases deliver the speed and scalability required for immediate insights.
- Machine Learning and AI: The success of AI models heavily relies on the ability to process and analyze vast datasets. Vector Databases provide the necessary infrastructure to efficiently train and deploy machine learning algorithms, enabling accelerated model training and inference.
- Genomic Research: Genomic data analysis demands immense computational power and storage efficiency. Vector Databases offer substantial benefits by enabling rapid analysis of genomic datasets, facilitating genome-wide association studies, variant calling, and personalized medicine research.
- Fraud Detection and Cybersecurity: Vector Databases play a crucial role in identifying and combating fraudulent activities. Their ability to process large volumes of data quickly and perform complex analytics enables the detection of anomalies and patterns indicative of potential fraud or security breaches.
These are just a few examples of the diverse applications of this kind of databases. Their unique capabilities make them indispensable in domains requiring high-performance analytics, real-time processing, and scalability. As organizations continue to face increasingly complex data challenges, Vector Databases provide a powerful solution to unlock valuable insights and drive innovation.
Comparison with Snowflake
While exploring Vector Databases, it’s important to draw parallels and distinctions with Snowflake Cloud Database, a widely adopted cloud-native data platform. Let’s examine how these two technologies align and differ.
Parallel 1: Cloud-Native Architecture Both Vector Databases and Snowflake leverage cloud-native architectures, allowing for seamless scalability, elasticity, and on-demand resource allocation. They harness the power of cloud infrastructure to handle massive workloads efficiently.
Parallel 2: Columnar Storage Both databases utilize columnar storage, a storage format that optimizes analytical workloads by storing data in columns rather than rows. This design facilitates faster data retrieval and improved query performance.
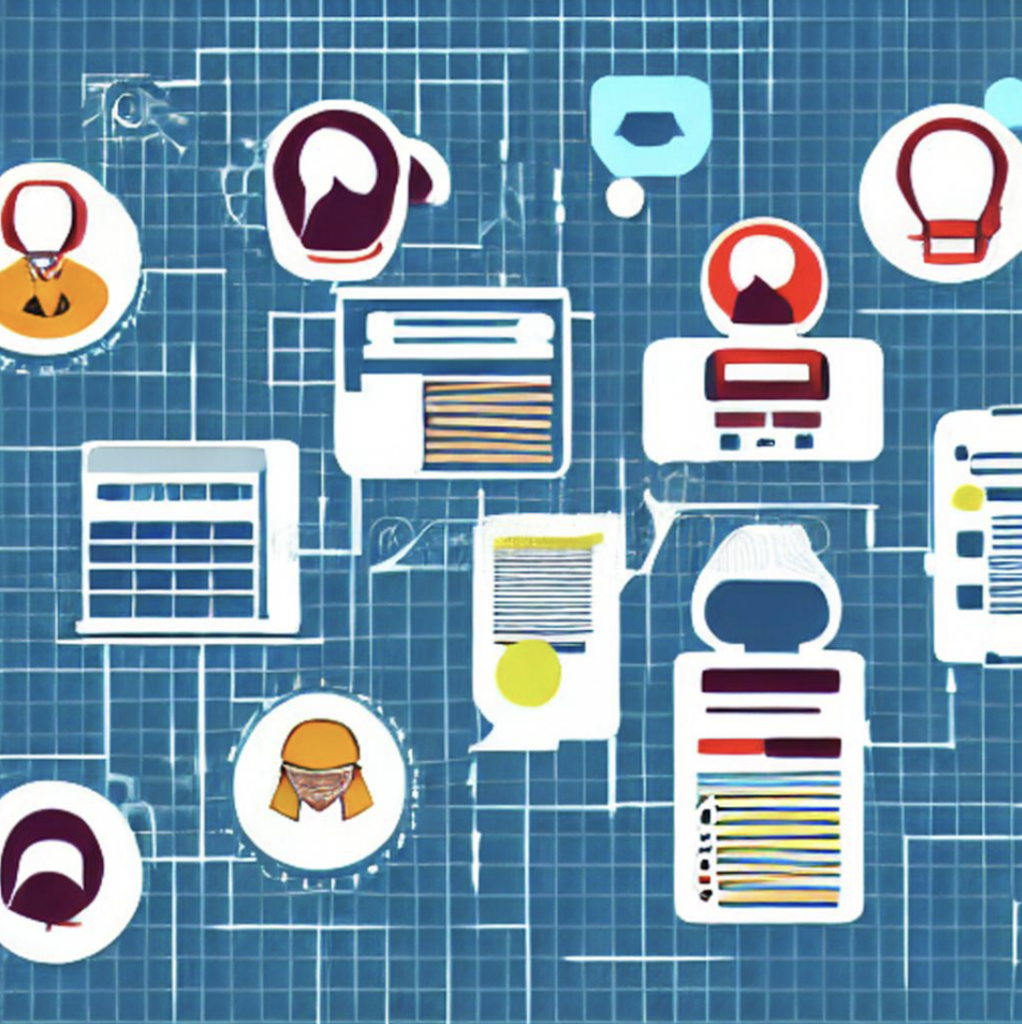
Difference 1: Vectorized Query Execution Vector Databases specialize in vectorized query execution, leveraging batch processing and SIMD instructions for enhanced speed and efficiency. In contrast, Snowflake employs a multi-cluster shared data architecture, offering parallelism through the distribution of workload across multiple compute clusters.
Difference 2: Workload Flexibility Snowflake provides a broad spectrum of workload capabilities, supporting diverse data processing needs, including transactional, analytical, and data engineering workloads. Vector Databases, on the other hand, focus primarily on analytical workloads and advanced analytics use cases.
By understanding these parallels and differences, organizations can evaluate the strengths of each technology based on their specific requirements. Whether it’s the vectorized processing power of Vector Databases or the versatile workload capabilities of Snowflake, choosing the right platform is essential for achieving optimal performance and scalability in the cloud.
Final thoughts
In this blog post, we embarked on a journey through the realm of Vector Databases. We uncovered their immense potential in the world of data analytics. This blog explored the fundamental concepts of vectorized query execution. On top, columnar storage, and SIMD were introduced, understanding how these technologies revolutionize data processing and analysis.
Further, this blog showed parallels and distinctions with the widely adopted Snowflake Cloud Database. The shared focus on cloud-native architectures and columnar storage was recognized, while also acknowledging the unique strengths of each platform.
As organizations grapple with the challenges of unstructured data and the accelerating progress of AI, Vector Databases emerge as a powerful tool to unlock valuable insights. From real-time analytics to machine learning and beyond, these databases offer the speed, scalability, and efficiency necessary to tackle complex analytical workloads.
By embracing Vector Databases, organizations can stay at the forefront of the data-driven revolution, empowering themselves with the capabilities to extract meaningful insights and drive innovation in today’s dynamic landscape.
Make sure to check out the other Blogs of Nimbus Intelligence!